Artificial intelligence (AI) has revolutionized numerous industries, and in 2025, financial forecasting stands as one of the key beneficiaries. By leveraging advanced algorithms and powerful data analytics, businesses can make informed financial decisions with greater precision. AI enables organizations to analyze vast datasets, identify patterns, and predict financial outcomes with improved accuracy. As financial markets become more complex, AI’s ability to process diverse information makes it a crucial tool for investment strategies, budgeting, and risk management.
Key AI Technologies for Financial Forecasting
Several AI technologies are essential for financial forecasting in 2025. Machine learning (ML) plays a pivotal role, using supervised learning models to predict outcomes based on historical data and unsupervised learning for pattern recognition. Deep learning (DL) excels in complex financial environments by analyzing intricate data structures through neural networks. Natural Language Processing (NLP) enhances market understanding by extracting insights from news, reports, and social media trends. Generative AI is valuable for creating simulated market scenarios to evaluate potential risks and opportunities. Reinforcement learning stands out in adapting investment strategies based on real-time market changes, ensuring automated systems continuously improve predictions. These technologies collectively form the backbone of modern financial forecasting, improving both accuracy and decision-making capabilities.
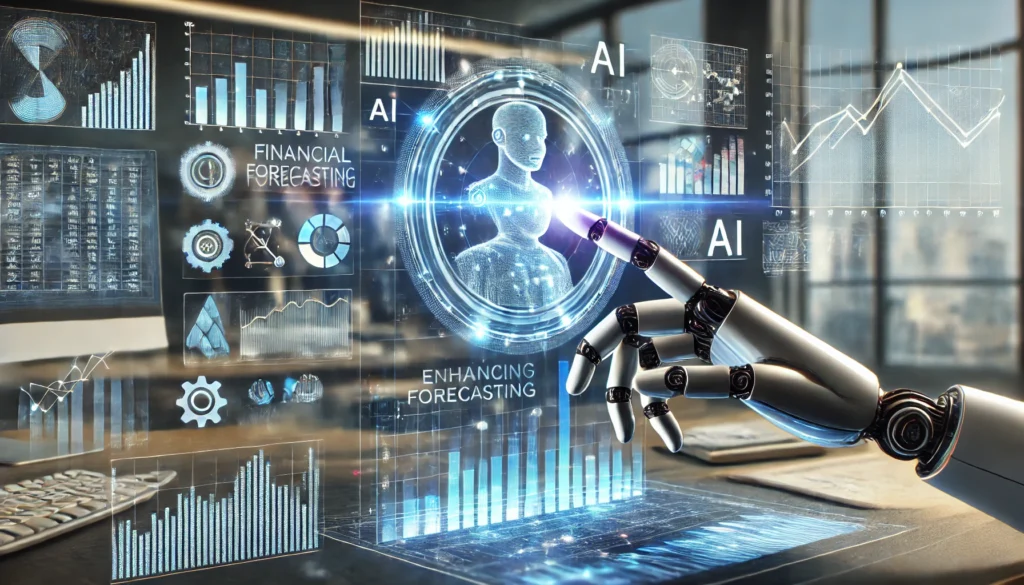
Steps to Implement AI in Financial Forecasting
Implementing AI for financial forecasting requires a structured approach. The first step is data collection, where historical financial data, economic indicators, and social-political trends are gathered to provide comprehensive insights. Next, data cleaning and preprocessing are crucial to remove noise, outliers, and inconsistencies, ensuring the data is reliable for AI models.
Model selection is vital, as choosing between ML, DL, or hybrid models depends on the dataset’s complexity and forecasting goals. Once selected, models must be trained and tested using cross-validation techniques to enhance their accuracy. Fine-tuning hyperparameters ensures models perform optimally under various conditions. Finally, deploying these models involves integrating them into financial systems, enabling real-time analysis, continuous monitoring, and performance evaluation to ensure sustainable results. Each stage demands precision, as errors in earlier steps can significantly impact forecasting accuracy.
Tools and Platforms for AI Forecasting
AI forecasting thrives on powerful tools and platforms. TensorFlow, PyTorch, and Scikit-learn are widely used frameworks that offer extensive libraries for building and refining predictive models. For data integration, financial data platforms such as Alpha Vantage, Yahoo Finance API, and Quandl provide essential data feeds for real-time insights.
Cloud solutions like Google Cloud AI, Azure AI, and AWS AI further enhance forecasting efficiency by offering scalable computing power and dedicated AI services. These platforms simplify model deployment, facilitate automated updates, and provide data security features. Selecting the right tools depends on data volume, forecasting objectives, and system compatibility. Combining flexible frameworks with trusted data platforms empowers businesses to build accurate, data-driven forecasts tailored to their financial goals.
Best Practices for Effective AI Forecasting
Effective AI forecasting relies on several best practices. Ensuring data quality is paramount; inaccurate data can drastically affect predictions. Regular data audits, cleaning, and normalization help maintain data integrity. Using explainable AI (XAI) ensures transparency by revealing how models generate predictions, boosting stakeholder trust. Combining AI insights with expert financial knowledge prevents over-reliance on automated outputs, enhancing overall accuracy.
Additionally, continuous model refinement is essential. As market conditions shift, AI models require regular updates to adapt and improve forecasting precision. Implementing real-time monitoring systems enables businesses to identify performance gaps and address potential issues promptly. Following these best practices ensures AI models deliver actionable insights that align with financial strategies.
Challenges and Risks in AI Financial Forecasting
While AI offers significant benefits, several challenges persist in financial forecasting. Data bias and inaccuracies can skew predictions, resulting in flawed investment decisions. Ethical concerns arise as automated systems influence financial outcomes, requiring businesses to uphold responsible AI practices. Regulatory compliance is another challenge, as financial authorities impose strict guidelines on data usage and predictive algorithms.
AI models are also vulnerable to overfitting, where excessive focus on past data reduces adaptability to future market changes. Furthermore, unpredictable global events can disrupt financial markets, making even the most advanced AI models struggle to maintain accuracy. Addressing these challenges requires robust data governance, risk management protocols, and a balance between automated insights and expert judgment.
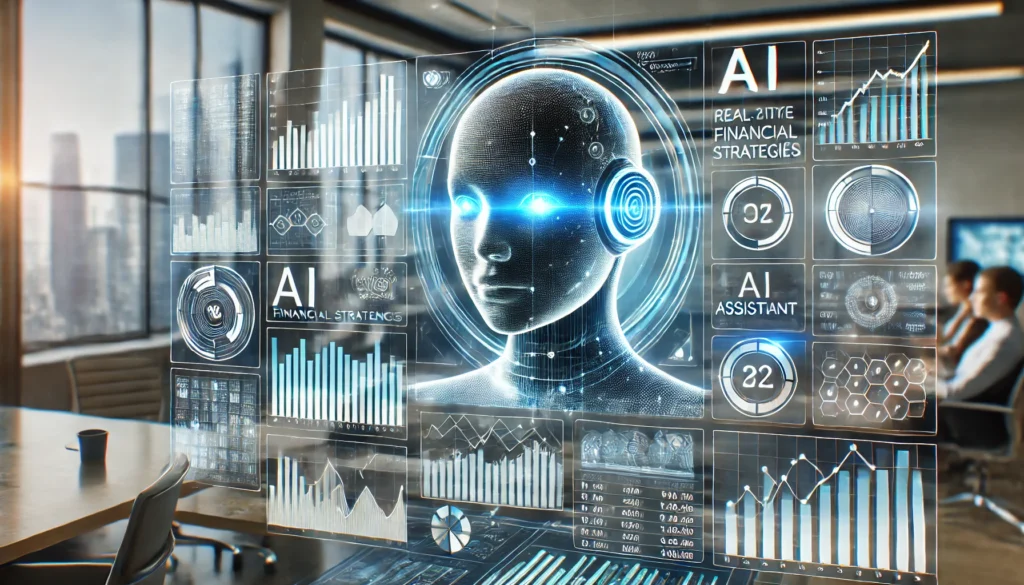
Future Trends in AI for Financial Forecasting
AI’s future in financial forecasting is set to evolve dramatically. Autonomous trading bots are gaining traction, utilizing AI algorithms to execute trades based on real-time market data. Enhanced sentiment analysis tools will leverage NLP to analyze media, social trends, and public perception to predict market movements. Blockchain integration is another significant trend, ensuring secure data exchange and improving transparency in financial reporting.
AI-powered virtual financial assistants are also emerging, offering personalized investment advice tailored to individual financial goals. As these innovations unfold, businesses must remain adaptable, adopting cutting-edge technologies to stay competitive in dynamic financial landscapes. The future of AI forecasting lies in blending automation with strategic expertise, unlocking new opportunities for financial growth.
Conclusion
AI has become an indispensable tool for financial forecasting in 2025. By leveraging machine learning, deep learning, and NLP technologies, businesses can improve forecasting accuracy and financial decision-making. Implementing AI models requires careful data preparation, model selection, and monitoring to ensure consistent results. While challenges such as data bias and regulatory concerns persist, businesses that follow best practices and adopt emerging trends will maximize AI’s potential. As financial markets continue to evolve, integrating AI into forecasting strategies will become essential for achieving sustainable growth and informed investment decisions.